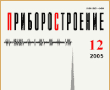
VECTOR REPRESENTATION OF WORDS USING THE APPARATUS OF QUANTUM PROBABILITY THEORY
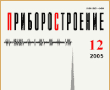
Annotation
The problem of modeling the semantics of text documents based on vector representation of words in a Hilbert space is considered. The vector representation of a word reflects the words surround-ing the given word (the context of the word). If a word is found in a document more than once, the set of contexts of a word forms its generalized context, i.e. meaning of the word. Different contexts of a word may be considered as different projections, and the generalized context — as a reconstructed multidi-mensional object. The purpose of the presented study is to improve the quality of the restoration of a word the context by considering additional factors, in particular, the possible non-orthogonality of con-texts. To achieve the goal, quantum probability theory is used here, and the context recovery procedure corresponds to the problem of quantum tomography in quantum physics. The task of restoring a word context or, in terms of quantum mathematics, the probability density matrix, is solved by the method of gradient descent using machine learning. Restrictions on the learning process are implemented by a set of regularizers that ensure the convergence of the process according to the Kullback—Leibler diver-gence criterion.
Keywords
Постоянный URL
Articles in current issue
- METHOD OF DIAGNOSTIC SYSTEM SYNTHESIS BASED ON BOOLEAN COMPLEMENT WITH REDUCED NUMBER OF CORRECTION ELEMENTS
- EXPERIMENTAL VERIFICATION OF A MATHEMATICAL MODEL FOR EFFUSION ANALYZER OF GAS VISCOSITY WITH DECREASING OUTCOMING PRESSURE
- SYNTHESIS OF A SUBORDINATE CONTROL SYSTEM WITH PREDETERMINED OVERSHOOT OF SERVO AXLE ELECTRIC DRIVE OF QUANTUM-OPTICAL COMPLEX
- DIGITAL TRACKING SYSTEM WITH FINITE DECAY TIME OF FREE PROCESS
- SPECIFIC OF USING MICROELECTRONIC COMPASSES IN COMPLEX NAVIGATION SYSTEMS
- DETERMINATION OF ACCURACY PARAMETERS OF MULTI-STAGED GEAR MECHANISMS
- METHODS OF THE HAND ELECTROMYOGRAM ANALYSIS
- METHOD OF MULTI-CRITERIA ANALYSIS OF WAREHOUSE PREMISES RENTING COST
- INDEX OF ARTICLES