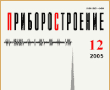
FORECASTING MULTI-SEASONAL LOAD PROCESSES IN ELASTIC COMPUTING SYSTEMS
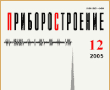
Annotation
The correctness of using the multi-seasonal season-trend decomposition method based on locally weighted scattergram smoothing for the problems of forecasting multi-seasonal load processes in elastic systems is assessed. A comparative analysis of the performance and accuracy of the above method and the seasonal integrated autoregressive moving average (SARIMA) model is performed. Results of experiments are presented that confirm the difficulty of constructing the SARIMA model based on data with a high degree of discretization and period values exceeding classical seasonality, such as 7, 12, 52. When creating the SARIMA model, time restrictions are imposed on the selection of parameters due to high memory consumption, which lead to a decrease in forecast accuracy and limited the ability to build a model based on higher seasonality indicators. The multi-seasonal season-trend decomposition method demonstrates an advantage over the SARIMA model in terms of forecast execution time and memory consumption, however, with a small set of initial data, the SARIMA model shows higher accuracy.
Keywords
Постоянный URL
Articles in current issue
- CHECKING THE AGREEMENT OF THEORETICAL AND EMPIRICAL PARETO DISTRIBUTIONS FOR COMPUTER COMMANDS AND MICROCOMMANDS USING THE KOLMOGOROV CRITERION
- METHODOLOGY FOR ASSESSING THE OPERATION SYSTEM OF COMPLEX OBJECTS BASED ON THE FUZZY-POSSIBILITY APPROACH
- REVIEW ON OPTIMIZATION TECHNIQUES OF BINARY NEURAL NETWORKS
- METHODOLOGY FOR EFFECTIVE COMPUTER MODELING OF A DEVICE FOR MEASURING LIGHT SCATTERING PROPERTIES
- ARITHMETIC CALCULATOR BASED ON NEURAL LOGIC ELEMENTS
- EFFECTIVE PHOTOSENSITIVE SURFACE OF SILICON PHOTOMULTIPLIERS
- CREATING CONTRAST-SUPPRESSED ABDOMINAL AORTA CT DATASETS FOR TRAINING AND TESTING ARTIFICIAL INTELLIGENCE ALGORITHMS
- EVALUATION OF THE STRENGTH OF ELASTOMERIC MEMBRANES OF PRESSURE CONTROL DEVICES