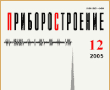
COMPARATIVE STUDY OF NEURAL NETWORK ARCHITECTURES FOR INTEGRATED SPEECH RECOGNITION SYSTEM
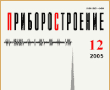
Annotation
The problem of improving the architecture of an integral neural-network model of Russian speech recognition is discussed. The considered model is created by combining the codec model with the attention mechanism, and the model based on the connectional temporal classification. Application of such neural network architectures as Highway Network, residual connections, dense connections, in the end-to-end model is studied. In addition, the use of the gumbel-softmax function instead of the softmax activation function during decoding is investigated. The models are trained using transfer learning method with English as non-target language, and then trained on a small corpus of continuous Russian speech with duration of 60 hours. The developed models are reported to demonstrate a higher accuracy of speech recognition in comparison with the basic end-to-end model. The results of experiments on recognition of continuous Russian speech are presented: the best result is 10.8% in terms of the number of incorrectly recognized characters and 29.1% in terms of the number of incorrectly recognized words.
Keywords
Постоянный URL
Articles in current issue
- METHODOLOGICAL AND METHODICAL BASES FOR CREATING AND USING INTEGRATED SYSTEMS OF DECISION-MAKING SUPPORT
- SOFTWARE AND MATHEMATICAL SUPPORT FOR COMPLEX OBJECTS MODERNIZATION
- FUZZY-PROBABILISTIC APPROACH TO FORMALIZING AND USING EXPERT KNOWLEDGE TO EVALUATE COMPLEX OBJECTS STATES
- METHOD FOR CONDUCTING SYNERGETIC OBSERVATIONS OF PROCESSES ON BOARD A SPACECRAFT
- INVESTIGATION OF STREAM CLUSTERING ALGORITHMS WHEN SOLVING THE PROBLEM OF SMALL SPACECRAFT TELEMETRY DATA ANALYSIS
- TECHNOLOGY OF AUTOMATED INFORMATION AND ANALYTICAL SUPPORT OF THE PRODUCT LIFE CYCLE ON THE EXAMPLE OF UNIFIED VIRTUAL ELECTRONIC PASSPORT OF SPACE FACILITIES
- VARIANT OF INFORMATION AND COMMUNICATION INFRASTRUCTURE BASED ON A CONTENT-CONTROLLED NETWORK
- COMPARATIVE STUDY OF NEURAL NETWORK ARCHITECTURES FOR INTEGRATED SPEECH RECOGNITION SYSTEM
- AUTOMATION OF LEGAL EXPERTISE OF AGREEMENT TEXTS
- FORMATION OF REQUIREMENTS FOR THE DESIGN PROCESS OF SECURE CYBER-PHYSICAL SYSTEMS
- EFFECTIVENESS OF DATA VISUALIZATION IN VIRTUAL REALITY