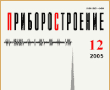
REVIEW ON OPTIMIZATION TECHNIQUES OF BINARY NEURAL NETWORKS
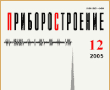
Annotation
The deployment of Convolutional Neural Networks (CNNs) models on embedded systems faces multiple problems regarding computation power, power consumption and memory footprint. To solve these problems, a promising type of neural networks that uses 1-bit activations and weights emerged in 2016 called Binary Neural Networks (BNNs). BNN consumes less energy and computation power mainly because it replaces the complex heavy convolution operation with simple bitwise operations. However, the quantization from 32-float point to 1-bit leads to accuracy loss and poor performance, especially on large datasets. This article presents a review of the key optimization techniques which influenced the performance of BNNs and led to higher representation capacity of BNN models, as well as an overview of the application methods of BNNs in object detection tasks and compares the performance with the real value CNN.
Keywords
Постоянный URL
Articles in current issue
- CHECKING THE AGREEMENT OF THEORETICAL AND EMPIRICAL PARETO DISTRIBUTIONS FOR COMPUTER COMMANDS AND MICROCOMMANDS USING THE KOLMOGOROV CRITERION
- FORECASTING MULTI-SEASONAL LOAD PROCESSES IN ELASTIC COMPUTING SYSTEMS
- METHODOLOGY FOR ASSESSING THE OPERATION SYSTEM OF COMPLEX OBJECTS BASED ON THE FUZZY-POSSIBILITY APPROACH
- METHODOLOGY FOR EFFECTIVE COMPUTER MODELING OF A DEVICE FOR MEASURING LIGHT SCATTERING PROPERTIES
- ARITHMETIC CALCULATOR BASED ON NEURAL LOGIC ELEMENTS
- EFFECTIVE PHOTOSENSITIVE SURFACE OF SILICON PHOTOMULTIPLIERS
- CREATING CONTRAST-SUPPRESSED ABDOMINAL AORTA CT DATASETS FOR TRAINING AND TESTING ARTIFICIAL INTELLIGENCE ALGORITHMS
- EVALUATION OF THE STRENGTH OF ELASTOMERIC MEMBRANES OF PRESSURE CONTROL DEVICES